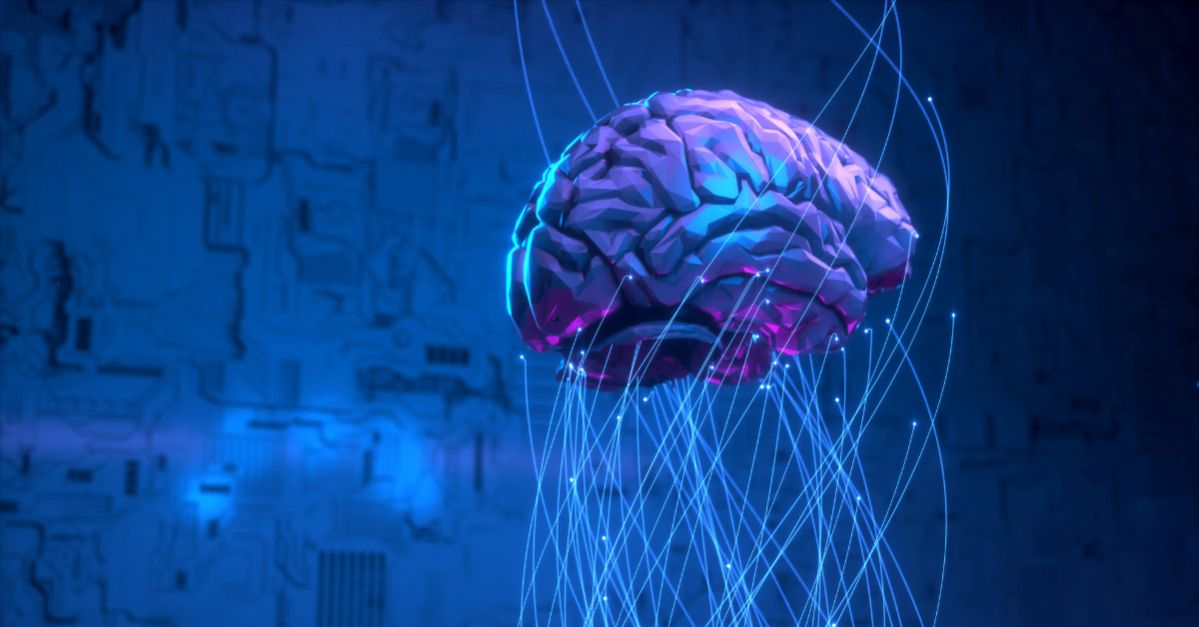
More and more organizations are eager to start using AI. But look a little closer, and you’ll see that many AI models don’t fail because of the technology – but because of the data they’re fed.
You want to forecast how much you’re going to sell. Plan smarter. Stay ahead of the competition. The tools are there. The models too. And yet, the results often don’t add up. Why?
Because the quality of your data simply isn’t good enough.
This article explores why data quality is the foundation of every AI initiative, where things typically go wrong in practice, and how a Data Quality Assessment can keep your AI project from failing before it even gets off the ground.
Where it goes wrong
In a BI environment, you can correct or compensate for a lot. But AI is unforgiving. A model learns from the data exactly as it is. And if that data is incomplete, inconsistent, or confusing, your predictions become unreliable.
Here are some examples we’ve encountered during assessments:
- Customer or supplier data with multiple spellings of the same name
- Incorrect address information
- Invalid email addresses
- Postal codes linked to the wrong countries
- Duplicate product records caused by variations in naming or classification (e.g. the same item listed under both 'Accessories' and 'Peripherals')
- Status fields with vague or inconsistent values
- Illogical combinations, such as mixing up installation date and manufacturing year
- Production waste entries without standard reasons or with free-text explanations
These kinds of inconsistencies can completely throw off an AI model. If you want to predict things like sales volumes, customer behavior, or machine failure costs, your underlying data needs to be clean and consistent.
What is a Data Quality Assessment?
A Data Quality Assessment doesn’t skim the surface — it takes a deep dive into your data:
- How many fields are empty, incorrectly filled in, or contain unrealistic values (outliers)?
- Are there duplicate or conflicting records? How logical is the structure and hierarchy?
- Is there inconsistency in how data is classified?
- Are there irregular patterns or anomalies that could mislead AI models?
We identify the weak spots and provide concrete advice: what can you improve now, what’s technically feasible, and how can you prepare your organization for reliable AI?
Why this step is often skipped – and why that’s a problem
As AI tools become more accessible, many organizations skip the foundation: data quality. They dive in with enthusiasm, only to realize later that their model is behaving unpredictably.
A Data Quality Assessment helps you stay ahead of that. It enables informed decisions, based on data you can actually trust.
AI starts with data. And that starts with control over its quality.
The author
Rik Smink
I help organizations develop AI solutions that accelerate processes, unlock opportunities, and enable future-focused decision-making.
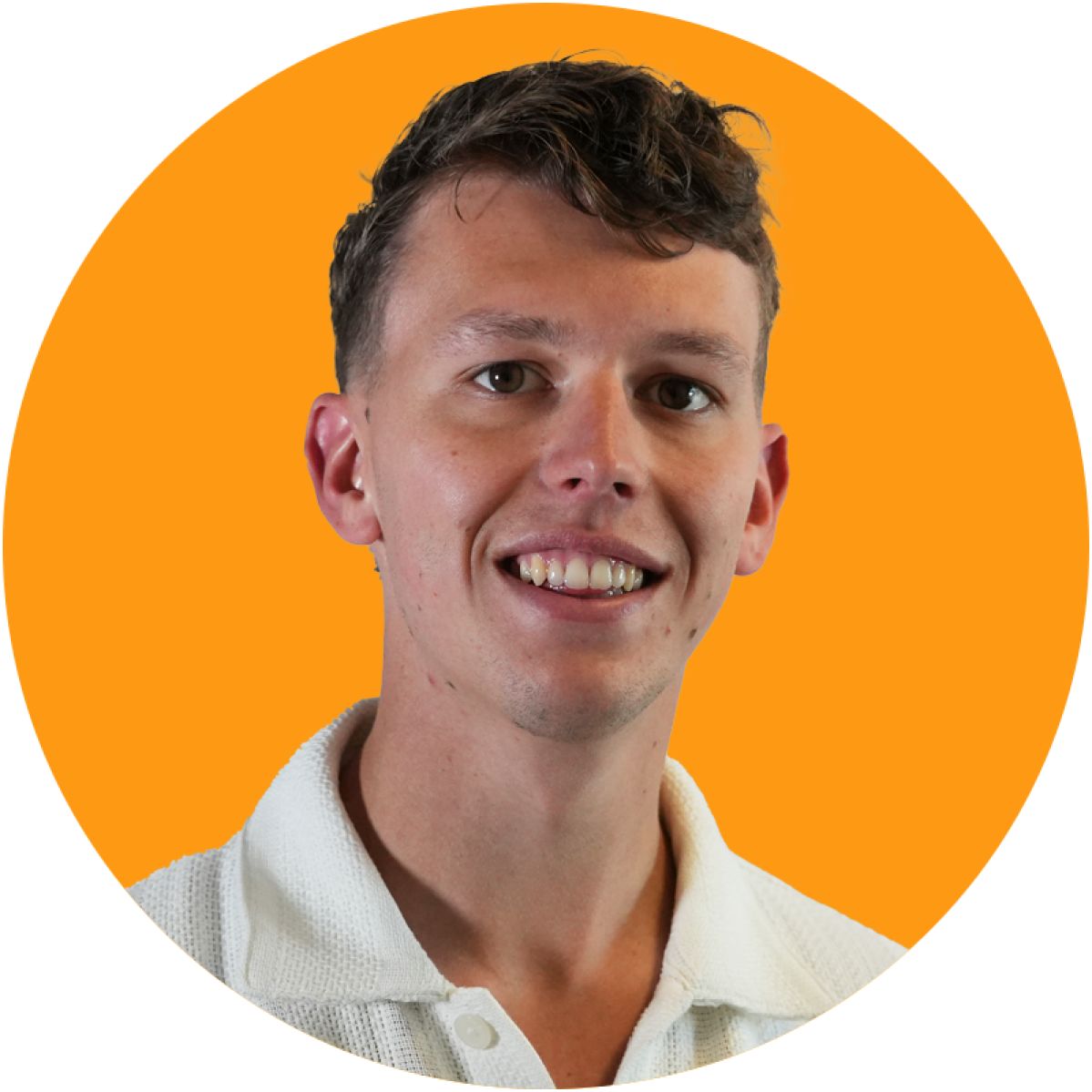